What is A/B testing?
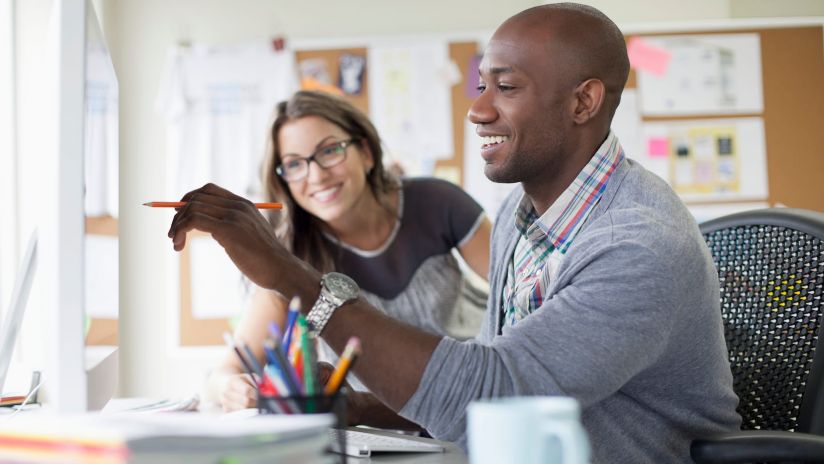
In its simplest form, A/B testing is a way to compare two versions of something to figure out which performs better. The principles and math behind A/B testing are around 100 years old.
Nowadays, A/B testing is most often associated with marketing and ecommerce. For example, testing different versions of websites or apps in an online, real-time environment.
Specifically, it involves creating two variations (A and B) that differ in one aspect. This could be a difference in design, layout, content or call-to-action. These variations are shown to similar groups of people, randomly selected from your target audience.
The importance of A/B testing
A/B testing is important for small businesses in several ways. It can help:
Optimise your marketing campaigns based on data
This helps boost conversions, such as more purchases. Making decisions based on data and real behaviour helps remove the guesswork or assumptions.
Provide insights into customer preferences and behaviours
Collecting this information can help tailor your offerings to better meet customer needs or expectations.
Deliver more cost-effective marketing
Sometimes businesses lack the resources or budgets and are looking for ways to find cost efficiencies. A/B testing helps by trying different variations without making significant investments.
How A/B testing works
A/B testing begins with knowing what you want to test. For example, this could be a call to action, such as the subscribe button on your website with two different colours. Importantly, only one variable should be tested at a time to get clear results.
Also consider how you’ll measure performance, for example conversion rates. For a subscribe button test, this is likely the number of people who click on the button.
To run the test, two sets of users, assigned randomly when they visit your website, are shown the different versions. Success is determined by which colour receives the most clicks.
Many factors can influence which colour people click on. For example, personal preference. This is where randomisation is crucial. It helps minimise the chances these factors affect your results.
A/B testing is often considered the most basic form of a randomised controlled experiment. As with all experiments of this kind, consider the sample size you need to gain statistical significance. This will help ensure the result isn’t due to other influences.
Some variables can impact the success metric. For example, it could be that mobile users spend less time on websites, compared with desktop users.
In performing an experiment, the randomisation process in one set (A) may contain slightly more mobile users than the other (B). As a result, that set may see a lower click-through rate regardless of the button size they’re seeing.
To help level the playing field, divide users by mobile and desktop and then randomly assign them to each version.
Common A/B testing scenarios
A/B testing can be applied in a variety of different ways to help better inform your digital marketing strategy and content creation. Below are some examples of where you can use A/B testing:
Website design and user experience
- Create different versions of a landing page to understand which leads to a higher conversion rate.
- Experiment with different fonts, colours, or layouts on your website or social media posts to see how each impacts user engagement.
- Test different versions of your site structure to see how user satisfaction is impacted.
Advertising campaigns
- Consider different copy, images or video content. This can help determine which version delivers more conversions.
- Create variations of landing pages or destination URLs. This can help you understand which improves post-click conversion rates.
Email marketing
- Test if different subject lines result in higher open rates.
- See how different call-to-action (CTA) buttons e.g. colour or size can help drive more clicks.
- Experiment with image placement in emails to see how it might help positively affect engagement.
Mobile apps
- Test different icons or app store descriptions to understand which helps increase app downloads.
- Experiment with different variations of in-app notifications or messages. This can help you understand which better drives your desired user actions.
- Create different user onboarding flows to see which helps improve user retention and engagement.
How to conduct A/B testing
If you want to run an A/B test, below is a step-by-step guide to help:
- Identify what you want to test.
- Define how you’ll measure the success of your A/B testing experiment e.g. click-throughs, conversion or time spent on a webpage.
- Create variations (A and B) and ensure there’s a single point of difference between them.
- Consider using A/B testing software. This can help set-up the experiment, evenly and randomly distribute your audience, and set a test duration.
- Run your test, track and collect your data.
- Analyse your data from both variations. Statistical analysis software can help you interpret results and decide which variation meets your goal.
- Decide if you wish to make changes and monitor performance.
How to interpret A/B testing results
Software can help you analyse A/B testing results. However, it’s helpful to have a basic understanding of the output to help you make decisions.
When interpreting survey results, it's helpful to consider margin of error and confidence levels. For example, your A/B testing may report 21% of people prefer a red call to action button. That result may have a margin of error of +/- 2% at 95% confidence.
But what does that mean? Margin of error is the measure of variability in your results based on your sample data. In this case our true result may sit between 19% and 23% of people who prefer a red call to action button.
A larger sample size generally results in a smaller margin of error. This is because it reduces sampling variability. Similarly, a smaller sample size may result in a larger margin of error.
Confidence levels indicate the level of certainty the results fall within the estimated range. Common confidence levels are 95% or 99%. This means that if you repeated your A/B testing many times, you would expect the result to fall within the margin of error 95 or 99 times out of 100.
A/B testing best practices
A/B testing is a quick and easy way to understand customer preferences and to help implement changes to your marketing initiatives. Below we list some tips to help enable a smooth process.
- Define clear objectives, know what you want to test and how you will measure success. Test only one variable at a time. There are other, more complex ways to measure multiple variables. However, A/B testing considers only one variable.
- Consider what sample size you need, based upon the margin of error you’re willing to accept.
- Run the tests for enough time to help gather the data you require.
- Use experts or software to help you interpret the results properly to help you make informed business decisions.
A/B testing is a simple, yet powerful tool to help you make the most of your digital presence. It helps turn assumptions into insights and uncertainties into opportunities. By comparing variations and analysing customer behaviour, you can make decisions based on data. This will help lead to improved conversion rates, better customer experience and greater success in meeting your business goals.
Reach your customers with online marketing
A strong online presence helps attract customers to your website. We can help you rank higher on search engines and to run online advertising campaigns, plus more.
Explore more on this topic
Grow your online presence with digital experts
Whether you need a website or a complete digital marketing strategy, we can help your business to thrive online.